Thank you, Carnegie Mellon University!
An expression of gratitude and summary of experience from one of Carnegie Mellon's latest graduates.

Table of Contents
This post serves as a thank you to all of the folks that have helped along the way to earning my Master’s degree including family, friends, faculty, staff, and fellow students. Without their support and encouragement, I would not have accomplished as much as I have. Before diving into the gratitude, first I explain why I chose to pursue a Master’s degree and how I chose my program.
1. Choosing Graduate School
Why Graduate School?
Choosing to return to school and enroll in a graduate program after working for a handful of years is a difficult decision to reach. I had established a rhythm working in professional life - would returning to academic/student life be worth the shift in lifestyle or short-term loss of earning potential? After about 2-3 years working fulltime, I realized that I needed to pursue higher education to work in the areas and domains that interest me. My previous role satiated a technical appetite, but lacked satisfaction in regards to quantatitive thinking and problem solving.
A friend recommended that I read about a growing field known as ‘data science’ and I encountered a great book by Zacharias Voulgaris, Data Scientist: The Definitive Guide to Becoming a Data Scientist (below, left). The book explores the growing data science field, different paths to becoming a data scientist, and how current data scientists describe the position. A key tool for depicting data science is Drew Conway’s Data Science Venn Diagram (below, right). The three sets are: hacking skills, math skills, substantive expertise. Prior to graduate school, I had strong technical and quantitative skills but lacked a true domain area of expertise.
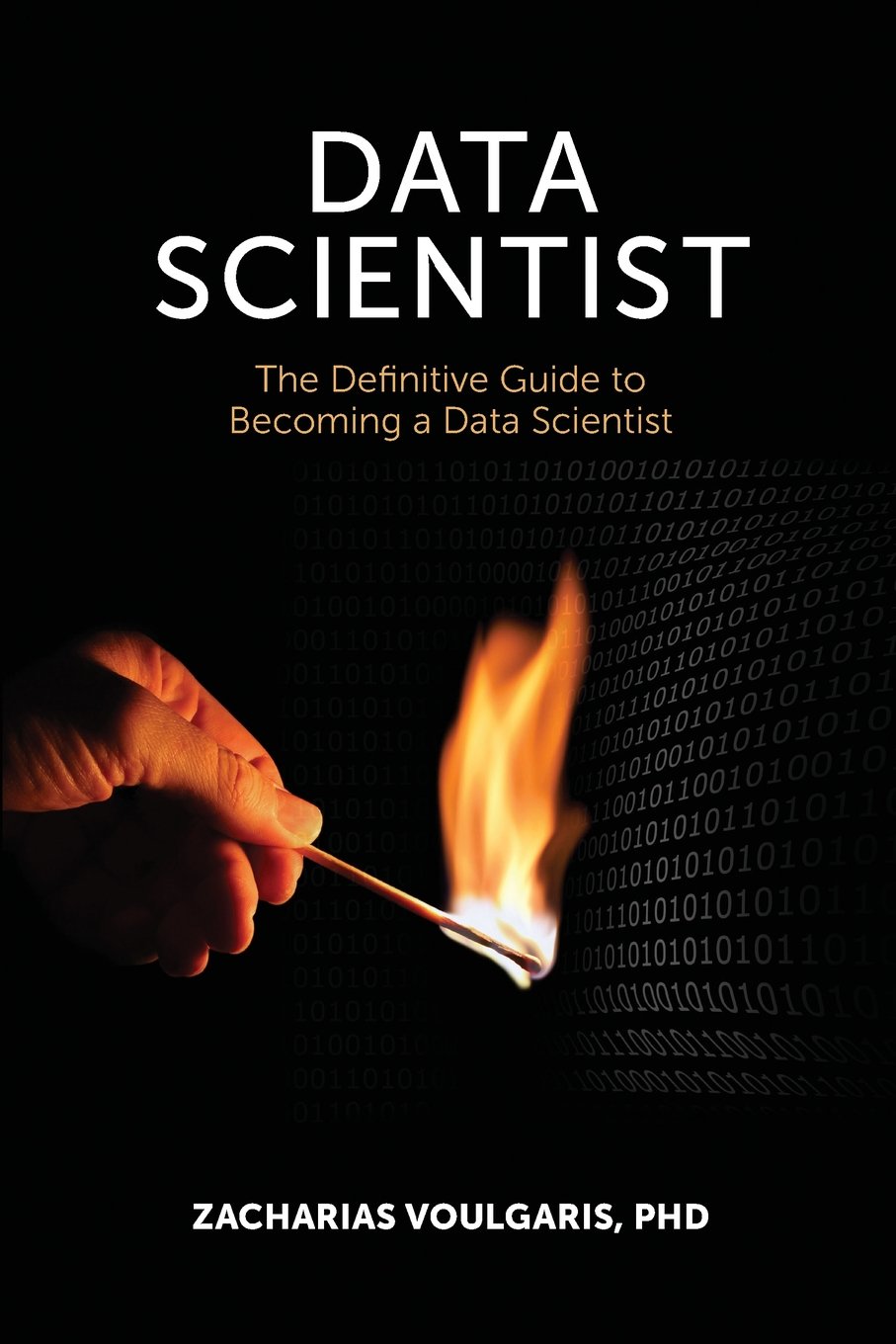
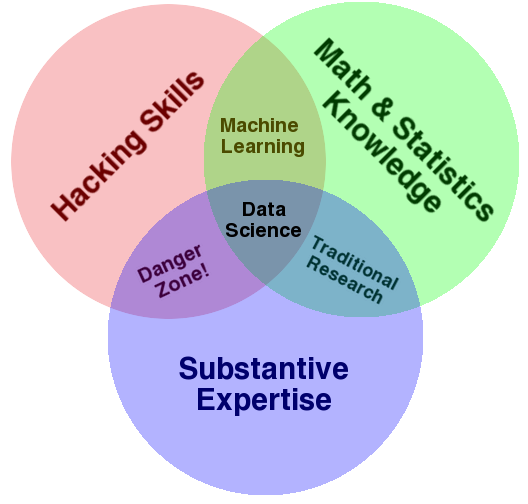
With a firm understanding of what data science is, and just as important, what data science will become during my professional career, I am convinced that data science and data-driven research is a perfect fit for me. Now, the decision to return to school was clear, the questions become: which school? which program?
Which Graduate School?
First, to answer the question of which graduate school to attend, I need to know in what domain area I am interested in applying data science and research methods. This question probes the important but often overlooked third set in Drew Conway’s Venn Diagram: substantive expertise. Especially as the data science field grows, much like in the information technology revolution of the late ‘90s and early ‘00s, specialization within the field will begin to take hold. In the data science case, this specialization could mean the tools that I use (Python, R, Stata, SAS, etc.) but much more likely refers to my domain area focus as this provides the highest marginal value, holding all else constant. Standard, rote memorization business problems often lack depth. What is more interesting is economic- and policy-focused analysis of large-scale national or international problems. Examples include healthcare policy, education policy, cybersecurity policy, technology policy, energy/environmental policy and economics/trade policy. The common theme is policy.
Given the policy focus derived above, I then need to understand what programs best align with my interests and can help fill all of the knowledge gaps that I have, while considering for reputation, job placement, proximity to home, etc. Fortunately, my research into graduate programs identified only a few top-tier programs that combine quantitative decision-making/analysis skills with robust data-focused technical skills. Ultimately, I found the Heinz College at Carnegie Mellon University which jointly houses the School of Public Policy with the School of Information Systems. Together, my coursework comprehensively covers the three requisite focus areas to become a proper data scientist as defined by Drew Conway’s Venn Diagram:
tech + quant + policy
2. Coursework
While the coursework is not the only component that defines the graduate student experience, and certainly not the only reason I am grateful for my experience (more on these other reasons to follow), it is a core component of a successful program. If the skills, techniques, and concepts that I hope to learn are not taught in an organized and thoughtful manner, then my time would be wasted. Fortunately, this was not the case in my program. As previously mentioned, the subjects are both broad and deep thanks to the full- and half-semester course structures at CMU.
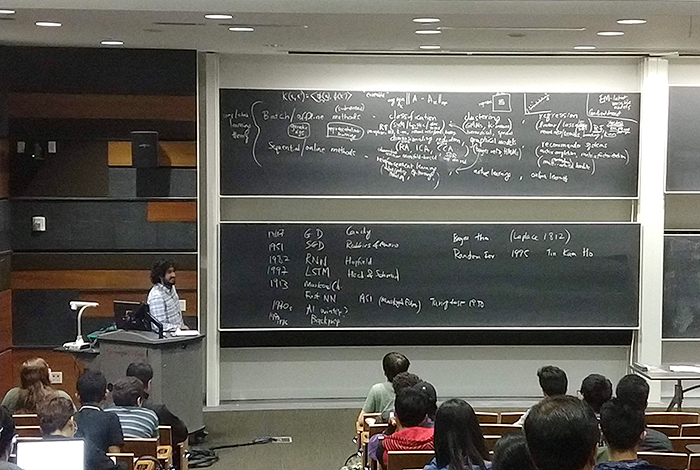
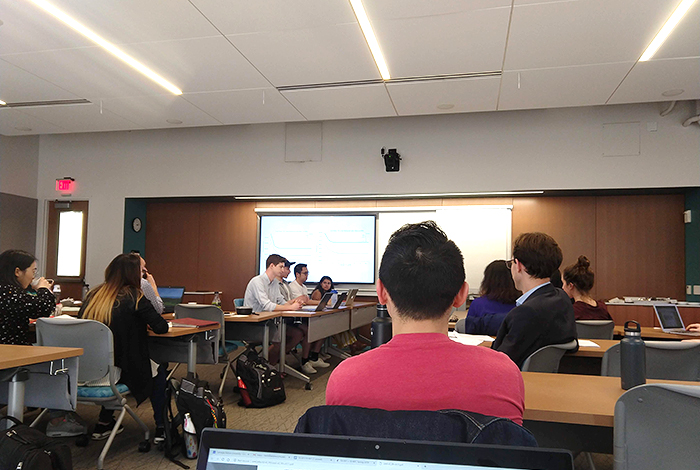
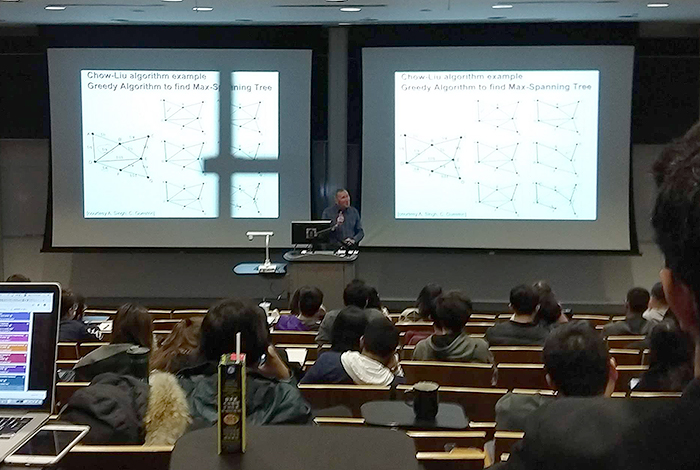
Machine Learning/Data Mining
- Data Mining
- Machine Learning
- Applied Analytics: The Machine Learning Pipeline
- Unstructured Data Analytics
- Data Science & Big Data
- Big Data & Large-scale Computing
Econometrics/Statistics
Management Science/Decision Optimization
Other Courses
- R Shiny for Operations Management
- Database Management
- Managing Analytics Projects
- Organizational Design & Implementation
- Analysis of Financial Statements
- Management Accounting
Teaching Assistantships
During the two-year program, I was fortunate to serve as a teaching assistant for four courses with four excellent professors/instructors:
- Data Focused Python (Spring 2018)
- Database Management (Fall 2018)
- Data Science & Big Data (Spring 2019)
- Big Data & Large-scale Computing (Spring 2019)
Some of my fellow teaching assistants (left: Database Management, right: Data Science & Big Data)...
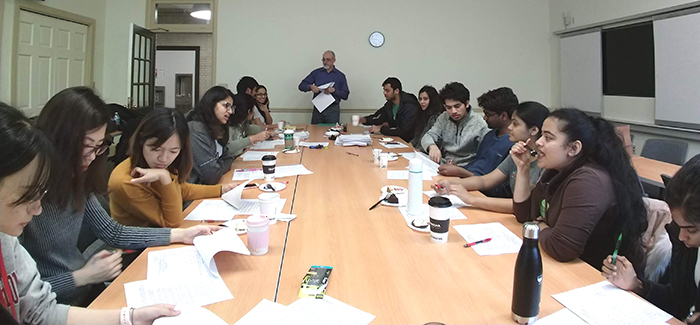
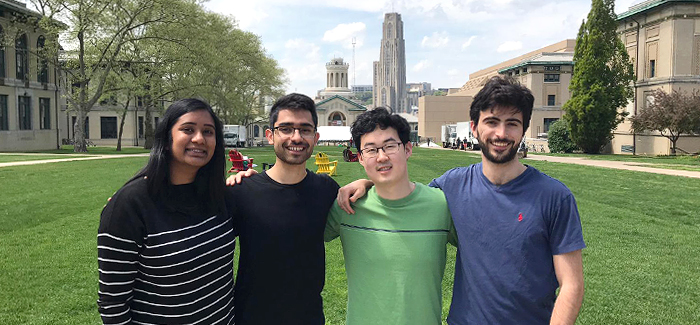
3. People
Professors/Instructors
Making the courses possible are, of course, the professors and instructors. Across the curriculum, I was impressed with their accomplishments, but more so impressed with their commitment to helping students learn, think critically about the subjects, and engage with the material inside and outside of the classroom. In alphabetical order by last name:
Advisors
- Karyn Moore, my academic advisor, helped me identify and sequence key courses to build a comprehensive, data-ready skillset.
- Diane Taylor, my career advisor, helped me identify potential career opportunities that align with my interests and navigate the job interview process.
Staff
There are too many excellent staff members to thank! Thank you to everyone who helped along the way from Academic Advising, Academic Services, Career Services, and Computing Services. I appreciate all of the help making sure I am aware of available courses, I can enroll in courses that I have a strong interest in taking, and I can also find space to work during peak demand.
Students
First and foremost, thank you to my fellow Data Analytics students! Second, thank you to all of the Heinz College student body - an engaging graduate student experience is not complete without incredible students. Lastly, thank you to all of the other CMU students that I was fortunate to interact with either academically or otherwise.
4. Grad Student Life
Campus & Study Space
The Carnegie Mellon campus and the Heinz College building (Hamburg Hall) are well maintained and recently renovated. It’s clear students were considered when buildings were designed. The university is highly accessible for students whether taking the CMU shuttle/bus or walking to campus. Finding a study room can be challenging during midterm or final exam weeks, but in general searching for a room is reasonable given the ample allocation of student study spaces. CMU’s Carnival provides a mostly-student-organized series of days with concerts, booths, petting zoos, carnival rides, and buggy races.
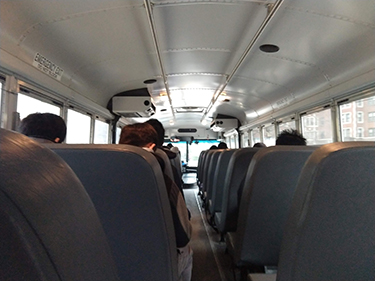
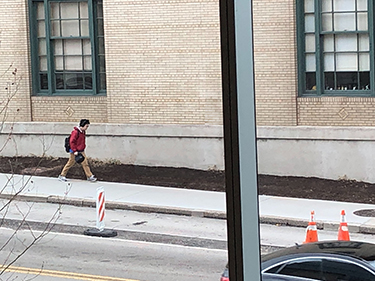
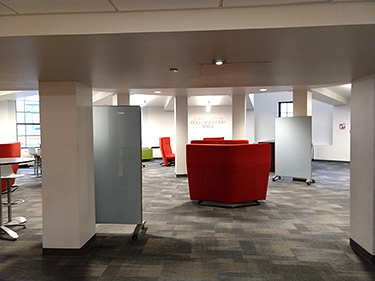
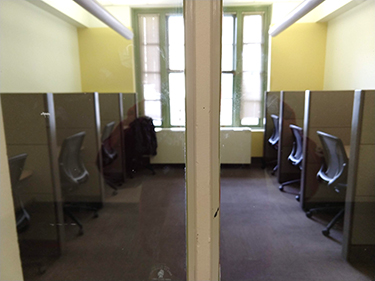
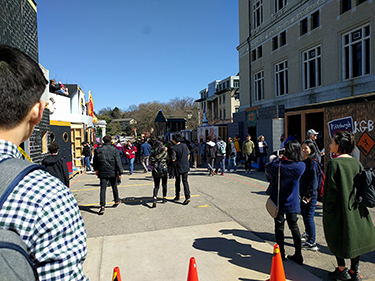
Seminars/Events
Collectively, the faculty, staff, and student body do an excellent job hosting events and seminars for continued learning outside of the classroom environment. Four examples are depicted below of events on topics spanning technology and policy research, negotiation and large-scale societal issues. Of course, there are many, many (…many…) more not shown. These seminars greatly enhance the graduate student experience because they build on the fundamental/core concepts that you learn and reveal the concepts’ direct applicability.
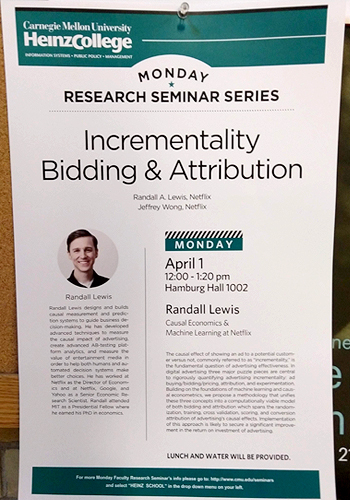
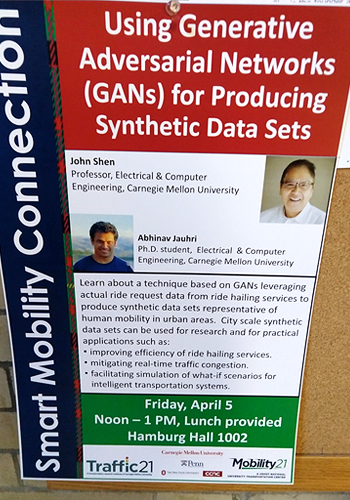
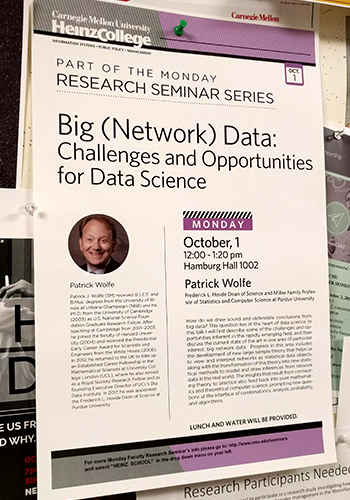
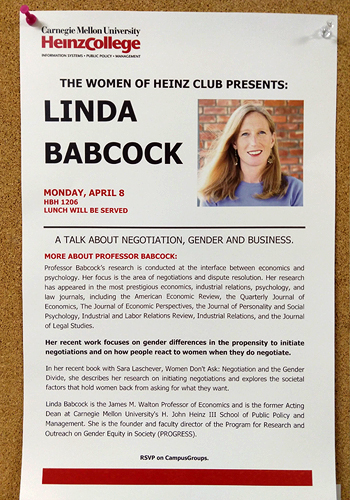
Student Organizations/Clubs
Student life at any level would not be complete without student organizations and clubs. As an undergraduate, I did not value the opportunity to augment my core curriculum as much as I do as a graduate student. The following clubs are a small sample of the clubs that are available across CMU’s campus, but these are the ones that I want to call out:
- Data Science Club is, as the name implies, a club that often has guest speakers from the data science industry, CMU faculty, or students who present on data science topics.
- MAD Tech Club focuses on building a campus-wide community surrounding innovative and disruptive technology including artificial intelligence (AI), surveillance, etc.
- Heinz Forum allows students to discuss current events affecting policy-making and trends in technology that will have a long-term societal impact. It is always good to hear opinions from your peers, no matter how wrong they may be!
Intramural Sports & Recreation
While enrolled, I enjoyed engaging with other graduate and undergraduate students in non-course related material by playing intramural soccer, intramural dodgeball, intramural kickball, and foosball. We finished with silver medals in the co-rec soccer and dodgeball leagues!
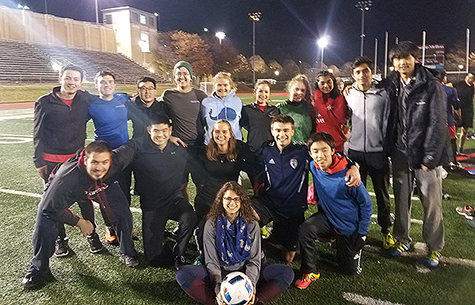
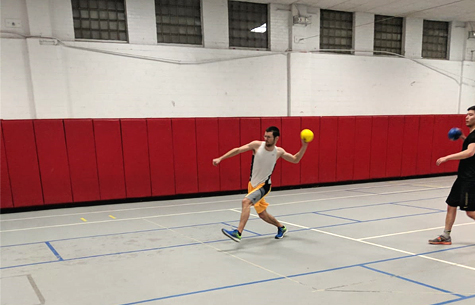
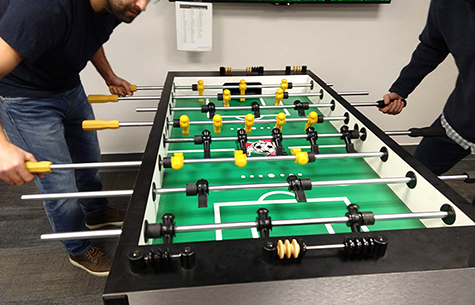
Internship
Using the skills I have developed in my coursework, I secured an incredible internship opportunity with the American Civil Liberties Union (ACLU) as a data scientist intern. I was able to apply R, Python, statistics, and machine learning to real-world, large-scale problems. You can read more about my Summer/Fall data science internship with the ACLU in their NYC headquarters here or by clicking the banner below.
5. Final Thoughts
With all of the metrics, loss functions, objective functions, and other ways of measuring performance for machine learning models, neural networks, data science methods, etc., it is imperative that a graduate student, especially one in a technically and quantitatively rigorous program, not forget how to define success for themself. As a fully-fledged data scientist about to embark on the next journey of my career, I constantly ask the question: What is my objective function? This line of thinking harks back to Drew Conway’s Venn Diagram (last time, I promise) and the importance of substantive expertise and domain knowledge. At some point, learning or devising your nth machine learning algorithm will no longer make you a better data scientist, if you are not passionate about one or more domain or substantive areas of expertise. In other words, if you are familiar with the explore/exploit paradigm often taught in the context of reinforcement learning, then you are exploring too much and never exploiting your technical and quantitative skills.
In my case, which may not generalize to others’ cases, policy is an area of interest for both data science and research. The opportunity to positively impact lives is maximized in this domain. Carnegie Mellon’s Heinz College, and its joint Schools of Information Systems and Public Policy, offers a unique opportunity for someone with my interest in data science, analytics, and public policy. Coursework in machine learning, decision optimization (management science), and, econometrics (searching for causality) plus other technical courses have prepared me to tackle nearly any given data set large or small, dense or sparse. I am planning to continue my career at a well-known consulting firm with a focus on data science and research with public organizations (governments, non-profits, etc.). If you are interested in learning more about my program or general CMU experience, please feel free to reach out. Thank you for reading about my experience!
Thank you, CMU!
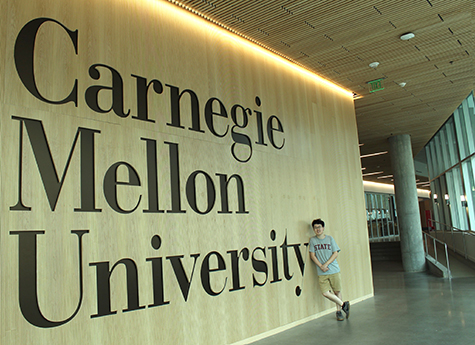
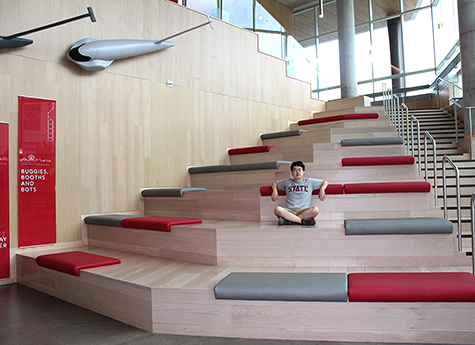
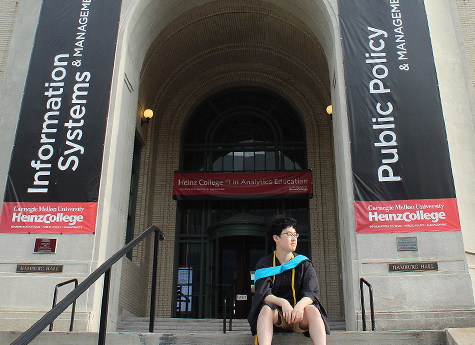
P.S. With my degree earned, I can finally get some much needed sleep.